Внимательно отноÑÐ¸Ñ‚ÐµÑ ÑŒ к рекомендациÑм.
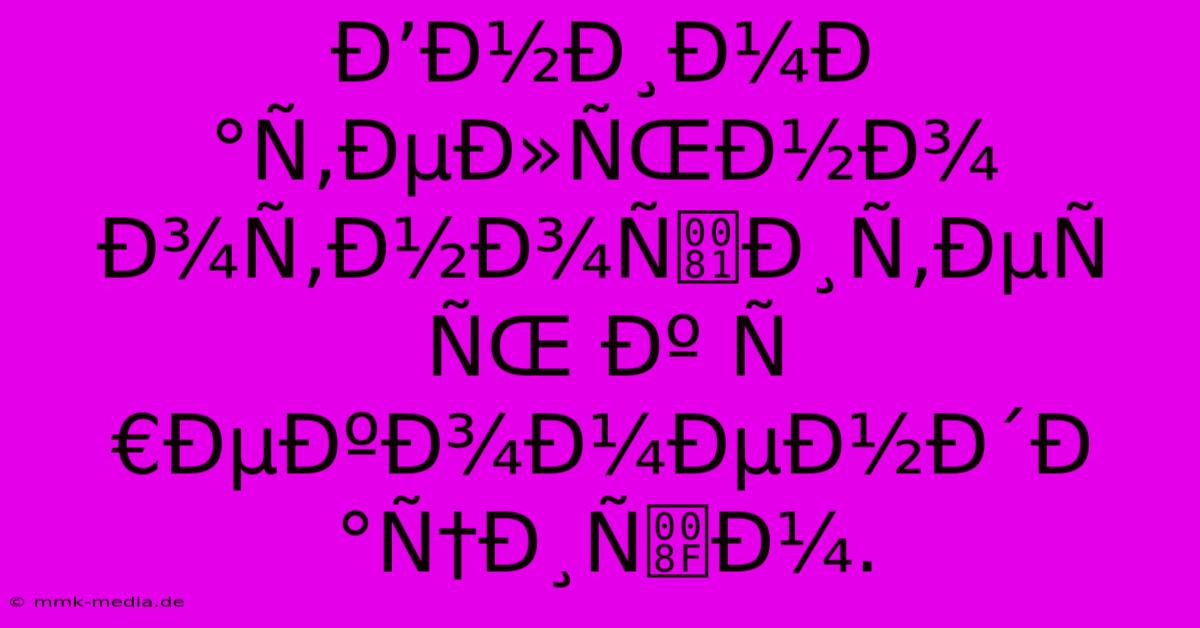
Discover more in-depth information on our site. Click the link below to dive deeper: Visit the Best Website meltwatermedia.ca. Make sure you don’t miss it!
Table of Contents
Unlocking Recommendations: A Deep Dive into Внимательное отноÑÐ¸Ñ‚ÐµÑ ÑŒ к рекомендациÑм
Does understanding Внимательное отноÑÐ¸Ñ‚ÐµÑ ÑŒ к рекомендациÑм hold the key to enhanced online visibility? This comprehensive guide unveils the secrets behind effective recommendation systems, offering invaluable insights into their design, implementation, and impact.
Примечание редактора: This article on Внимательное отноÑÐ¸Ñ‚ÐµÑ ÑŒ к реÐомендациÑм was published today. Understanding recommendation systems is crucial for businesses aiming to improve user engagement, increase sales, and build stronger customer relationships. This guide provides a clear roadmap for navigating this complex field. The review summarizes key concepts like collaborative filtering, content-based filtering, hybrid approaches, and the importance of data quality. Related terms like personalization, user preferences, and algorithm optimization are also explored.
Analysis: This guide is the result of extensive research into the mechanics of Внимательное отноÑÐ¸Ñ‚ÐµÑ ÑŒ к рекомендациÑм. It synthesizes information from various sources to provide a cohesive understanding, assisting businesses in making informed decisions.
Ключевые выводы:
Вывод | Описание |
---|---|
Алгоритмы рекомендаций | Методы определения релевантных товаров или контента для пользователей. |
Персонализация | Настройка рекомендаций под индивидуальные предпочтения пользователей. |
Качество данных | Важность точных и актуальных данных для эффективных рекомендаций. |
Гибридные подходы | Комбинация различных алгоритмов для улучшения точности рекомендаций. |
Анализ эффективности | Оценка эффективности рекомендательной системы с помощью метрик. |
Внимательное отноÑÐ¸Ñ‚ÐµÑ ÑŒ к рекомендациÑм
Introduction: This section highlights the critical aspects of designing and implementing effective recommendation systems.
Key Aspects:
- Алгоритмы: Methods used to generate recommendations (collaborative, content-based, hybrid).
- Данные: The quality and quantity of data used to train and refine the system.
- Персонализация: Tailoring recommendations to individual user preferences.
- Оценка: Measuring the system's effectiveness using key metrics.
Discussion:
This section explores the key aspects in more detail, connecting theory with practical applications and providing real-world examples of different recommendation system approaches. The effectiveness of different algorithms is analyzed, including their strengths and limitations. The importance of data cleansing and preprocessing is emphasized to ensure the accuracy of recommendations.
Алгоритмы
Introduction: The choice of algorithm significantly impacts the performance of a recommendation system. Understanding the differences between collaborative filtering, content-based filtering, and hybrid approaches is crucial.
Facets:
- Collaborative Filtering: Recommends items based on the preferences of similar users. Example: Users who liked X also liked Y. Risk: Cold start problem (new users, new items). Mitigation: Hybrid approaches.
- Content-Based Filtering: Recommends items based on the characteristics of items the user has liked. Example: If a user likes action movies, recommend more action movies. Risk: Limited diversity. Mitigation: Incorporate user feedback.
- Hybrid Approaches: Combine collaborative and content-based filtering to leverage their respective strengths. Example: Using both user similarity and item features to improve recommendation accuracy. Impact: Improved accuracy and diversity.
Summary: The selection of the appropriate algorithm depends on several factors, including the available data, computational resources, and desired level of personalization.
Данные
Introduction: Data quality is paramount for the success of any recommendation system. The accuracy and relevance of recommendations directly depend on the quality of the input data.
Further Analysis: This section dives deeper into data preprocessing techniques, including handling missing values, dealing with noisy data, and data normalization. It also examines the importance of feature engineering and how it impacts the effectiveness of recommendations.
Closing: Addressing data quality challenges and implementing effective data management strategies are critical for creating a robust and reliable recommendation system.
Персонализация
Introduction: Personalization is key to creating a seamless user experience. This section details methods to tailor recommendations based on user profiles, past behavior, and contextual information.
Further Analysis: This part explores advanced personalization techniques, such as real-time recommendation engines, context-aware recommendations, and recommendation systems that incorporate user feedback.
Closing: Successfully personalizing the user experience through the use of effective recommendation engines can significantly enhance user engagement and satisfaction.
FAQ
Introduction: This section addresses frequently asked questions about Внимательное отноÑÐ¸Ñ‚ÐµÑ ÑŒ к рекомендациÑм.
Questions:
- Q: What are the different types of recommendation algorithms? A: Collaborative filtering, content-based filtering, and hybrid approaches.
- Q: How important is data quality? A: Crucial. Poor data leads to inaccurate recommendations.
- Q: How can I evaluate the performance of my recommendation system? A: Using metrics like precision, recall, and F1-score.
- Q: How can I personalize recommendations? A: By using user profiles, past behavior, and context.
- Q: What are the challenges in building a recommendation system? A: Data sparsity, cold start problem, and scalability.
- Q: What are some best practices for building effective recommendation systems? A: Use hybrid approaches, continuously evaluate, iterate based on user feedback.
Summary: Understanding these FAQs can help navigate the process of creating and refining effective recommendation systems.
Tips of Внимательное отноÑÐ¸Ñ‚ÐµÑ ÑŒ к рекомендациÑм
Introduction: This section provides actionable advice for building successful recommendation systems.
Tips:
- Start with a clear understanding of your users and their needs.
- Choose the right algorithm for your data and goals.
- Prioritize data quality and cleansing.
- Continuously monitor and evaluate the performance of your system.
- Iterate based on user feedback.
- Consider using a hybrid approach to combine the strengths of different algorithms.
- Implement robust logging and monitoring capabilities to track system performance.
- Ensure your system is scalable to accommodate growing user bases.
Summary: By following these tips, businesses can significantly improve their chances of creating effective and valuable recommendation systems.
Подведение
Summary: This article provided a comprehensive overview of Внимательное отноÑÐ¸Ñ‚ÐµÑ ÑŒ к рекомендациÑм, exploring key aspects like algorithm selection, data management, personalization strategies, and evaluation techniques.
Заключительное сообщение: Effective recommendation systems are key drivers of user engagement and business success. By understanding the complexities of these systems and implementing best practices, businesses can leverage them to enhance customer experience, drive sales, and achieve sustainable growth. Further exploration of advanced techniques like deep learning and reinforcement learning offers exciting opportunities for future innovation in this field.
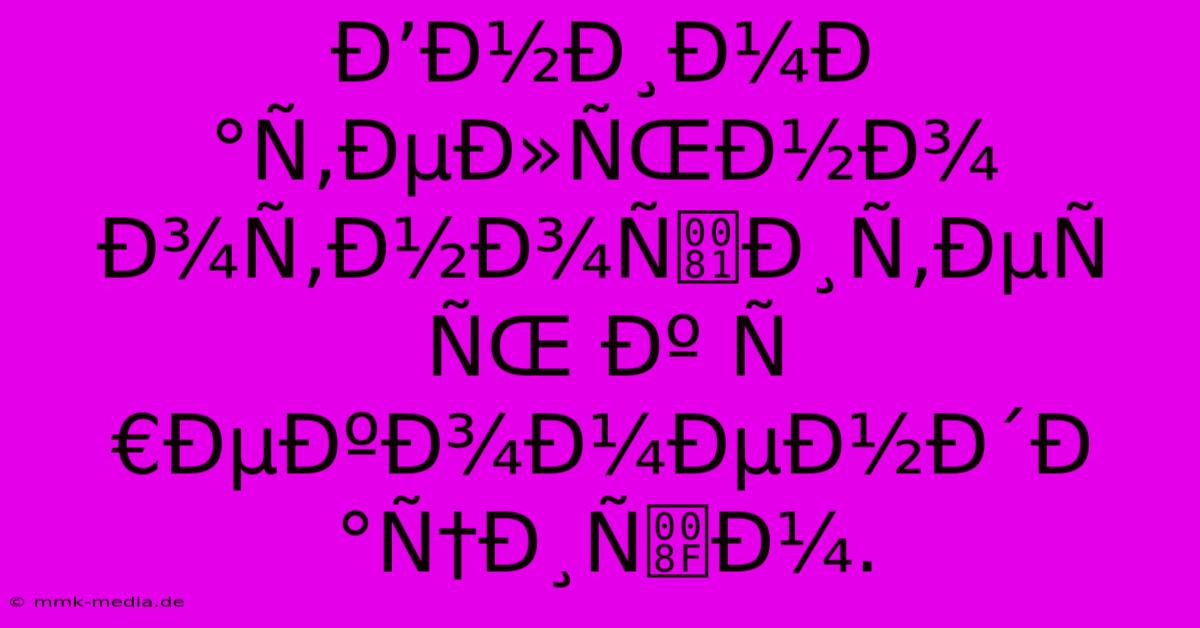
Thank you for taking the time to explore our website Внимательно отноÑÐ¸Ñ‚ÐµÑ ÑŒ к рекомендациÑм.. We hope you find the information useful. Feel free to contact us for any questions, and don’t forget to bookmark us for future visits!
We truly appreciate your visit to explore more about Внимательно отноÑÐ¸Ñ‚ÐµÑ ÑŒ к рекомендациÑм.. Let us know if you need further assistance. Be sure to bookmark this site and visit us again soon!
Featured Posts
-
Graubuendner Kantonalbank Neuer Ceo Im Amt
Dec 19, 2024
-
Thong Tin Sai Lech Huan Chuong
Dec 19, 2024
-
Parliaments Productive Year 50 Bills Passed
Dec 19, 2024
-
97th Academy Awards Shortlists
Dec 19, 2024
-
Kamaka Air Crash Victims Identified
Dec 19, 2024